EfficientGenAI inference
Build and scale production applications on the latest optimized models and fine tunes
Text Gen API
LLMs for chat, summarization, and structured output
Media Gen API
Diffusion models for stunning image and video
OctoStack
Turnkey GenAI stack in your environment
Innovators Choose OctoAI
“Working with the OctoAI team, we were able to quickly evaluate the new model, validate its performance through our proof of concept phase, and move the model to production. Mixtral on OctoAI serves a majority of the inferences and end player experiences on AI Dungeon today.”
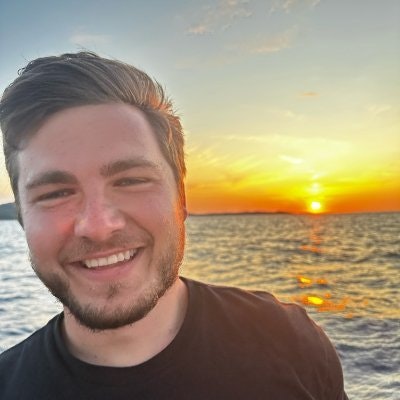
Nick Walton
CEO & Co-Founder Latitude
GenAI production stack: SaaS or in your environment
The foundation of OctoAI is systems and compilation technologies we’ve pioneered, like XG Boost, TVM, and MLC, giving you an enterprise system that runs in our SaaS or your private environment.
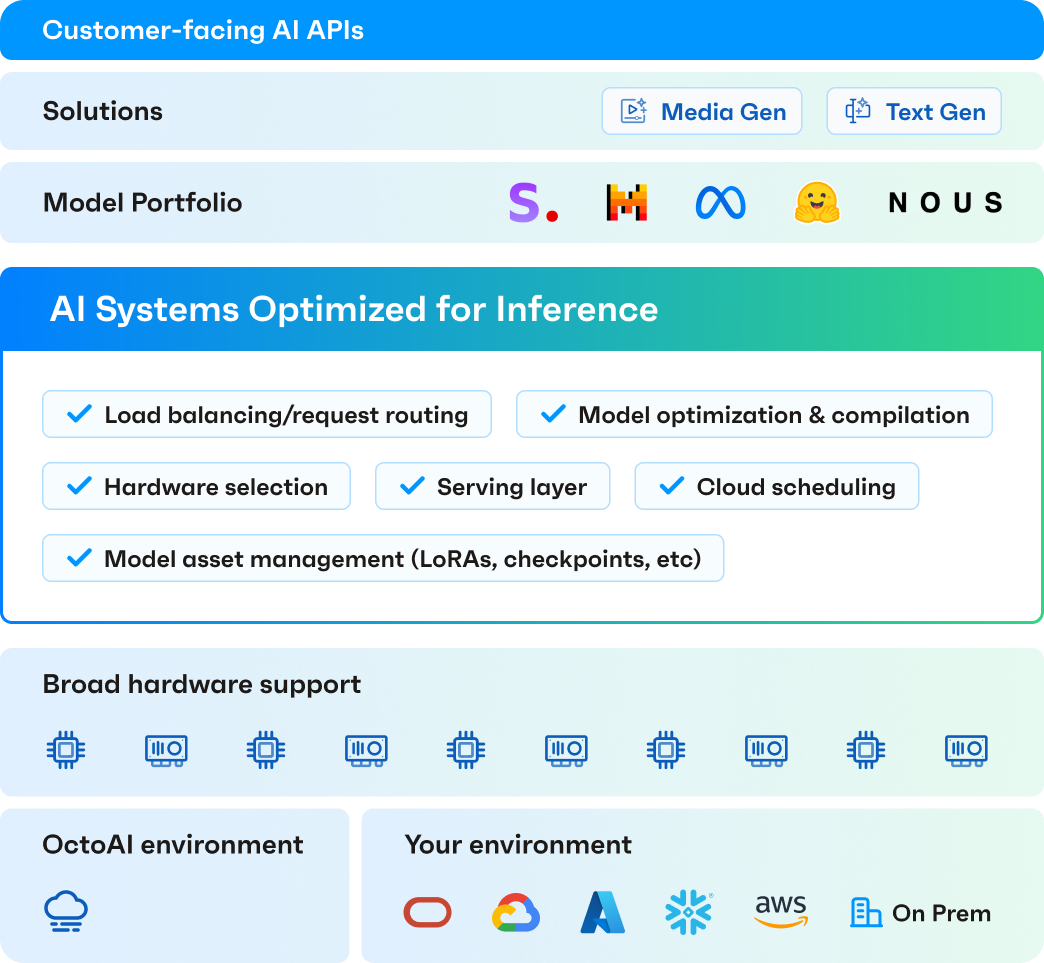
Enterprise-grade inference
Achieve AI Independence
Free yourself from any single model, model provider, cloud, or hardware setup.
Optimize Performance & Cost
Run GenAI inference at the lowest price and latency on our optimized serving layer.
Future Proof Applications
Rapidly iterate with new models and infrastructure without rearchitecting anything.
Customize Freely
Mix and match models, fine tunes, and AI assets at the model serving layer.
OctoStack from OctoAI: GenAI in your environment
OctoStack is a turnkey GenAI serving stack to run your optimized models in your environment on your GPUs. Lower your total cost of ownership and deploy models with greater agility while ensuring data privacy.

What’s New at OctoAI
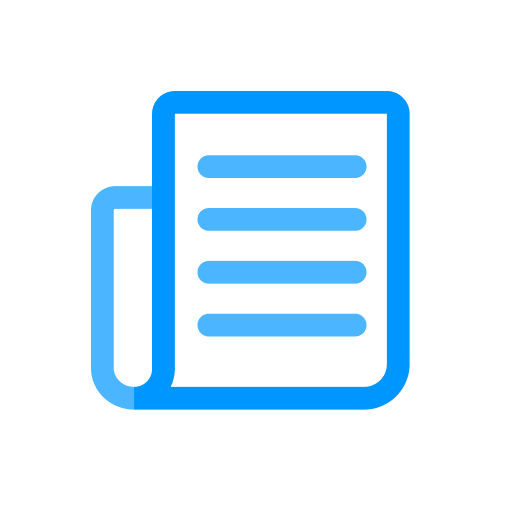
Customer & Product Updates
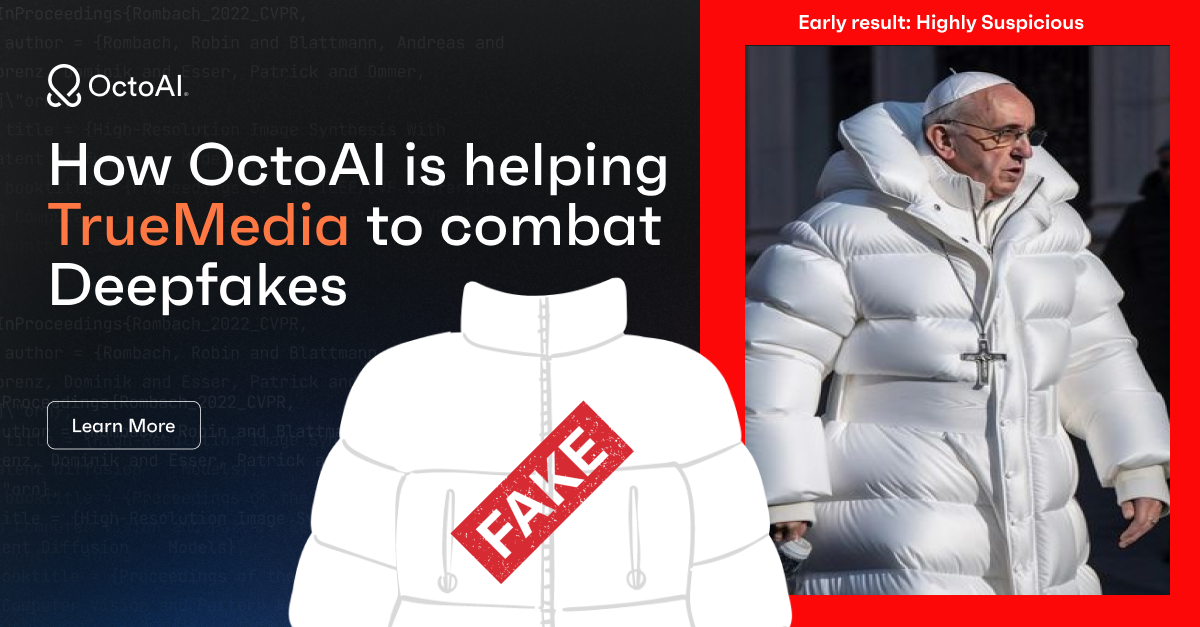
How OctoAI is Helping TrueMedia to Combat Deepfakes

Acceleration is all you need (now): Techniques powering OctoStack's 10x performance boost

NightCafe Studio: Pioneering AI-Driven Art Creation Through Community Engagement

Get the Most from Your Data with OctoStack and Snowflake
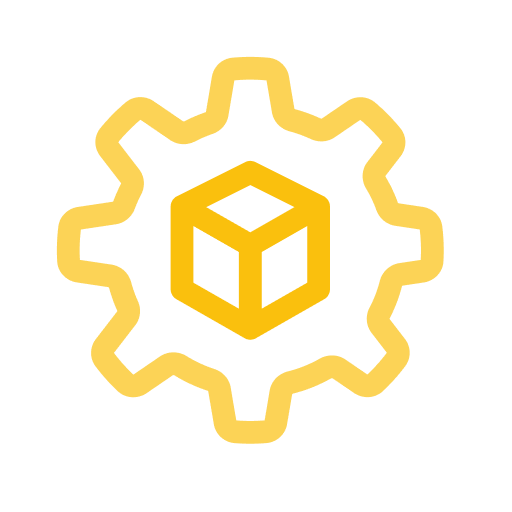
Latest Models
Llama 3 Instruct
Mixtral-8x22B Instruct
Mixtral 8x22B fine-tuned
Qwen 1.5
Your choice of models and fine tunes
Start building in minutes. Gain the freedom to run on any model or checkpoint on our efficient API endpoints.
%shell octoai asset create --name checkpoint-panda --upload-from-hf-repo NeuralNovel/Panda-7B-v0.1 \
--engine text/mistral-7b-instruct \
--data-type fp16 \
--format safetensors \
--type checkpoint \
--transfer-api sts